Blogs
Insights and innovations in AI Visual Inspection

The Role of Machine Vision in Manufacturing for Improved Quality Control and Assurance
Understand the crucial role of machine vision in manufacturing for enhanced quality control and assurance and learn why it's essential for modern manufacturing.
Thank you! Your submission has been received!
Oops! Something went wrong while submitting the form.
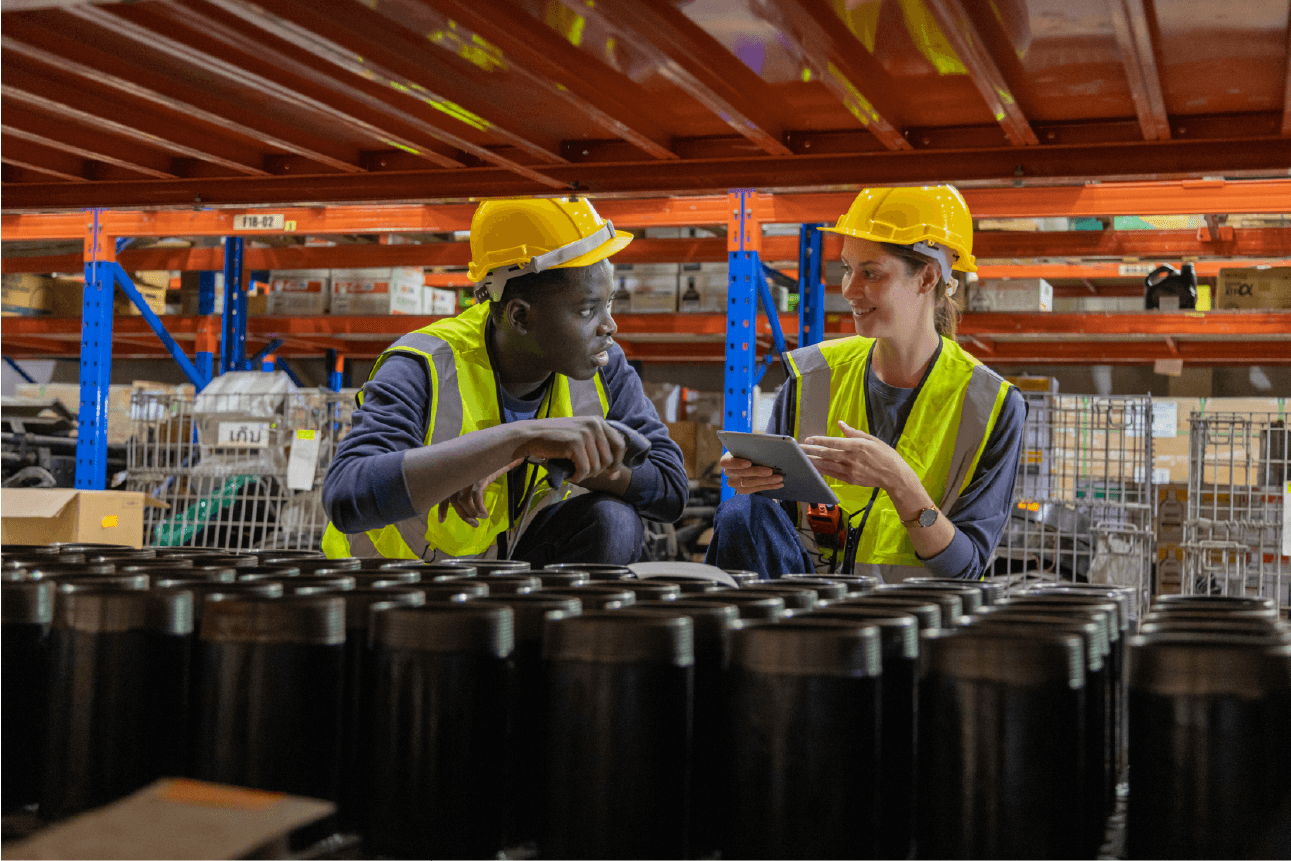
How AI and Real-Time Data Improve Traceability in Manufacturing
Explore how AI-powered visual inspection and real-time data are reshaping traceability in manufacturing. See how Loopr AI enables smarter, faster, and more reliable production workflows.
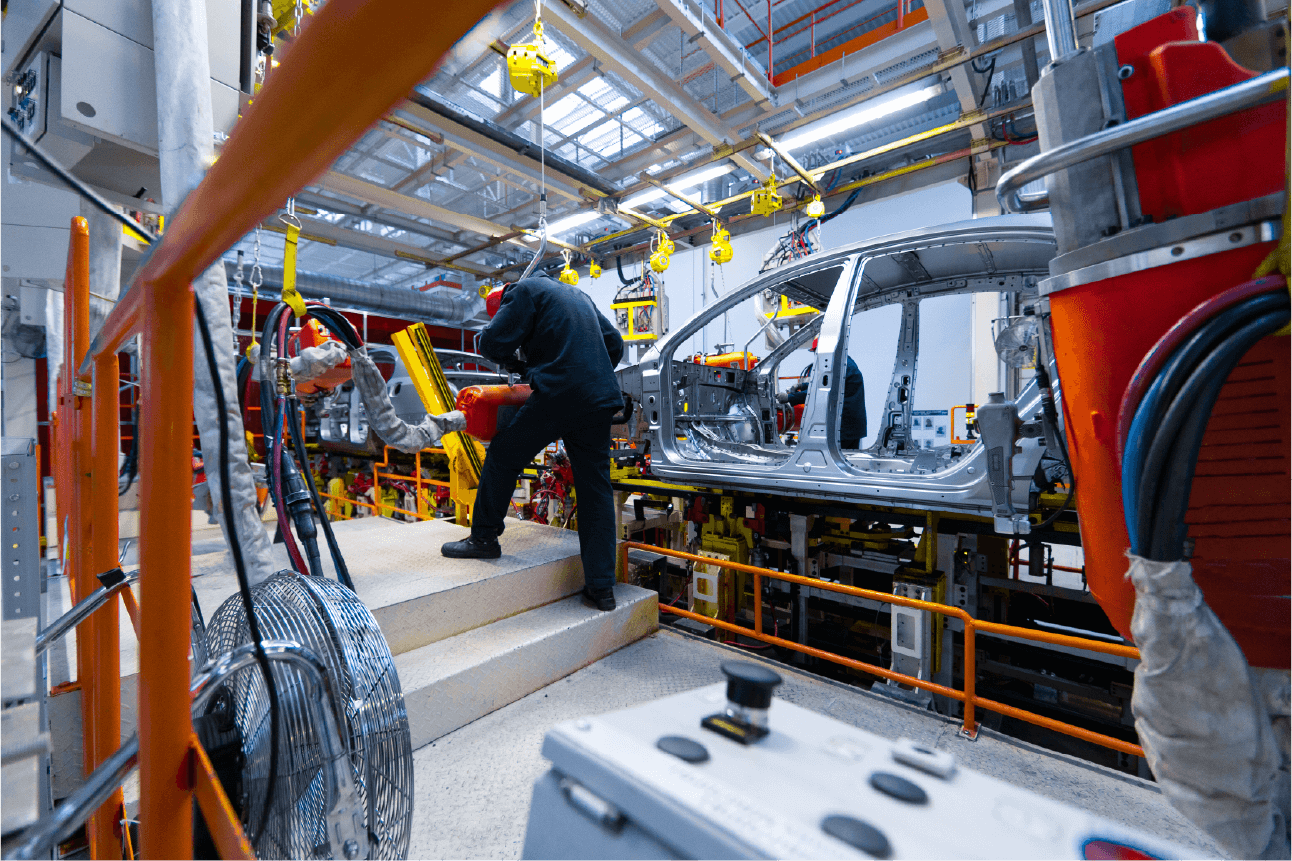
5 Manufacturing Challenges and How AI is Solving Them
Explore how AI is addressing some of the most important challenges in manufacturing quality

What is Smart Manufacturing? A Guide to Industry 4.0 Technologies
Learn more about Smart Manufacturing and Industry 4.0 technologies

Quality Assurance in Manufacturing: A Complete Overview
Explore what is quality assurance in manufacturing is and how AI-driven tools like visual inspection and defect detection are transforming modern QA.
.png)
Vision Inspection Systems: A Strategic Investment or Luxury?
Is automated vision inspection system worth it? Find out how AI-driven inspection systems enhance manufacturing quality, reduce waste, and optimize costs.

Transforming Quality Control in the Medical Industry with Machine Vision AI
Learn how Machine Vision AI is reshaping quality control in the medical industry by improving defect detection, compliance, and manufacturing efficiency.
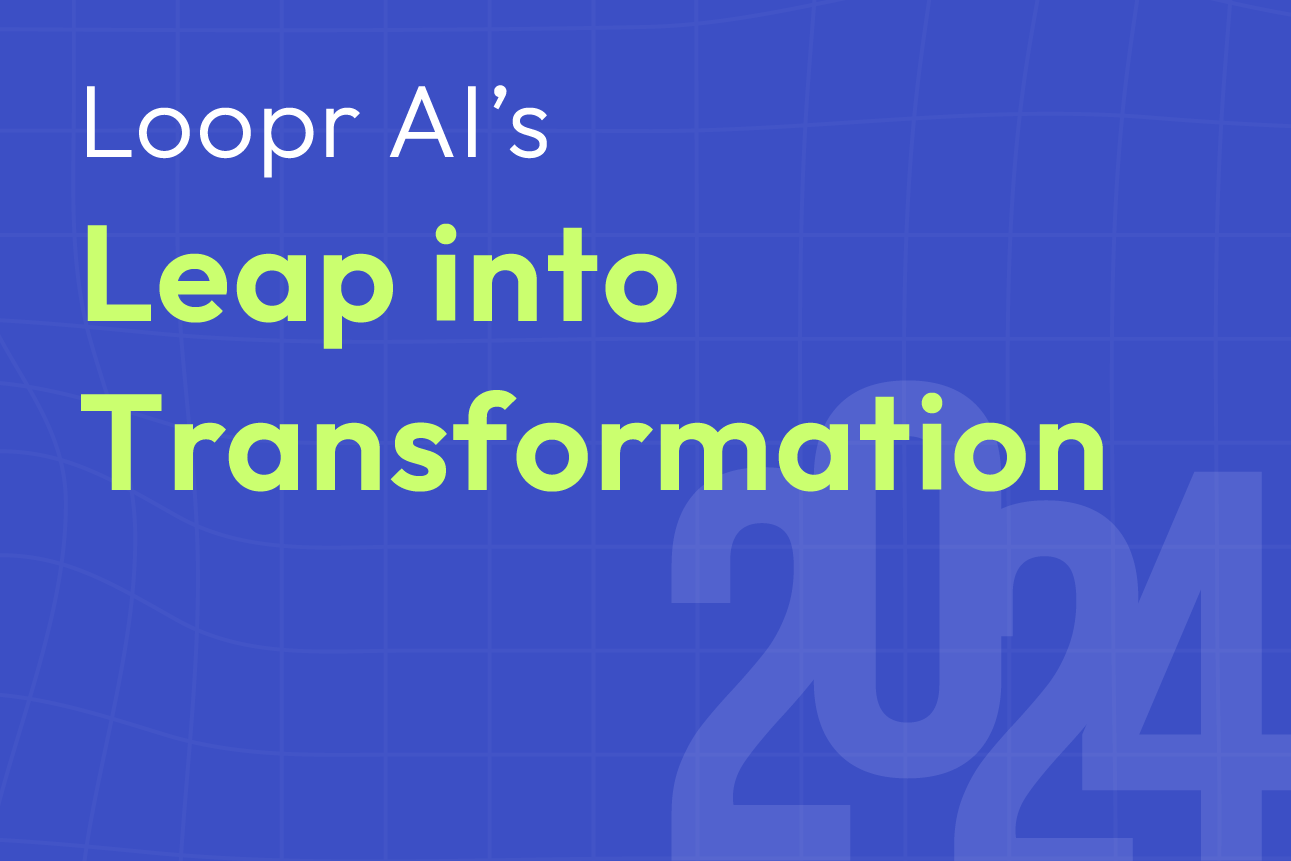
2024: Loopr AI’s Leap into Transformation
Loopr AI’s 2024 highlights include award-winning AI solutions, major milestones, and transformative innovations shaping aerospace and manufacturing.

Collaborative Intelligence: How AI and Humans Can Empower the Workforce Together
Understand how collaborative intelligence merges AI and human skills to boost productivity, innovation, and workforce efficiency across various industries.
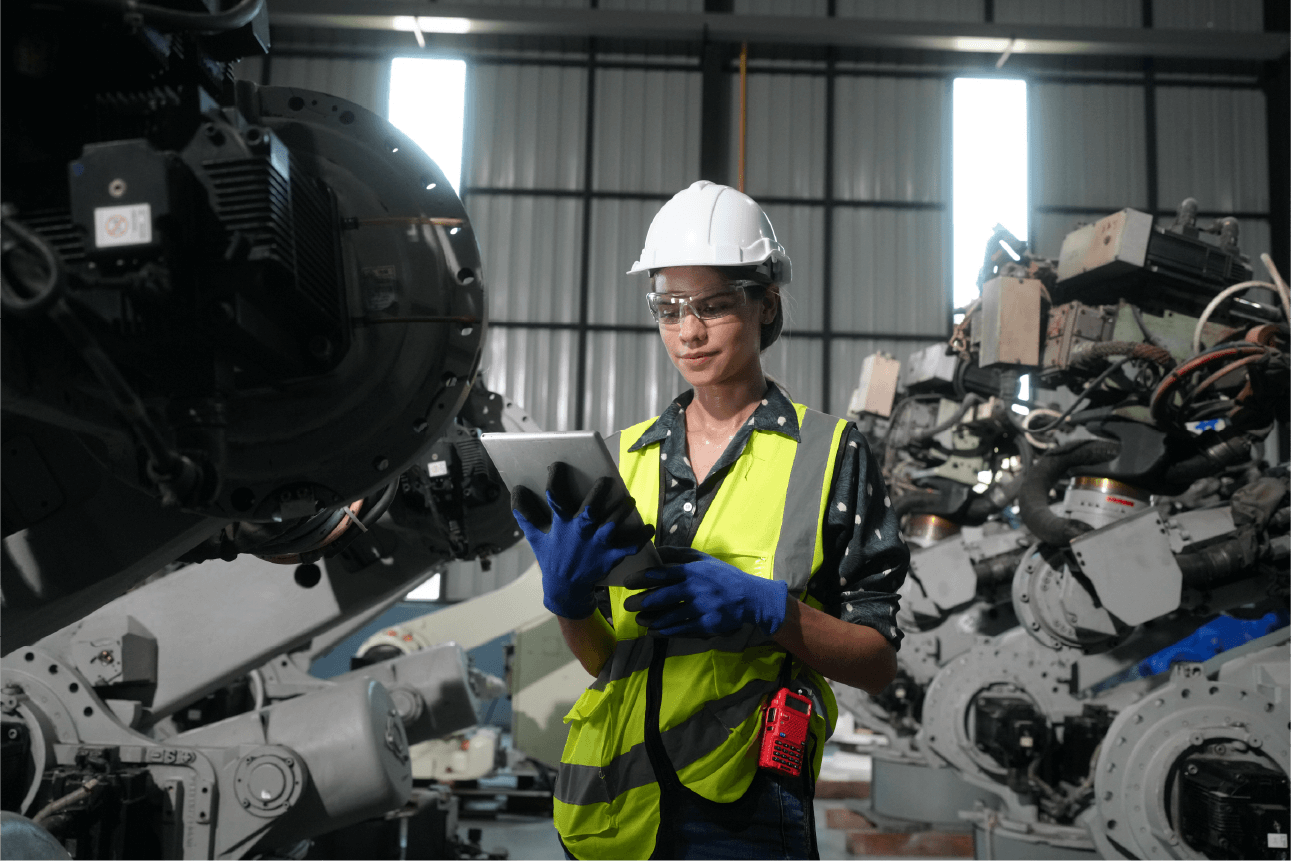
Quality Control Methods: Improving Accuracy with Real-Time Visual Inspections
Learn how modern quality control methods with AI-driven solutions improve accuracy, reduce defects, and enhance efficiency in manufacturing processes.
.jpeg)
Challenges in Aerospace Part Inspection in Manufacturing and How Machine Vision AI is Overcoming Them
Explore how Machine Vision AI transforms aerospace part inspection for unmatched precision and reliability
%202.jpeg)
How AI Visual Inspection is Transforming Quality Control in Manufacturing
Learn more about how AI visual inspection can improve quality control in manufacturing.

The Assembly Process: Everything You Need to Know
Explore everything about the assembly process, from manual to automated methods, key technologies, and tips to optimise manufacturing for quality and efficiency.
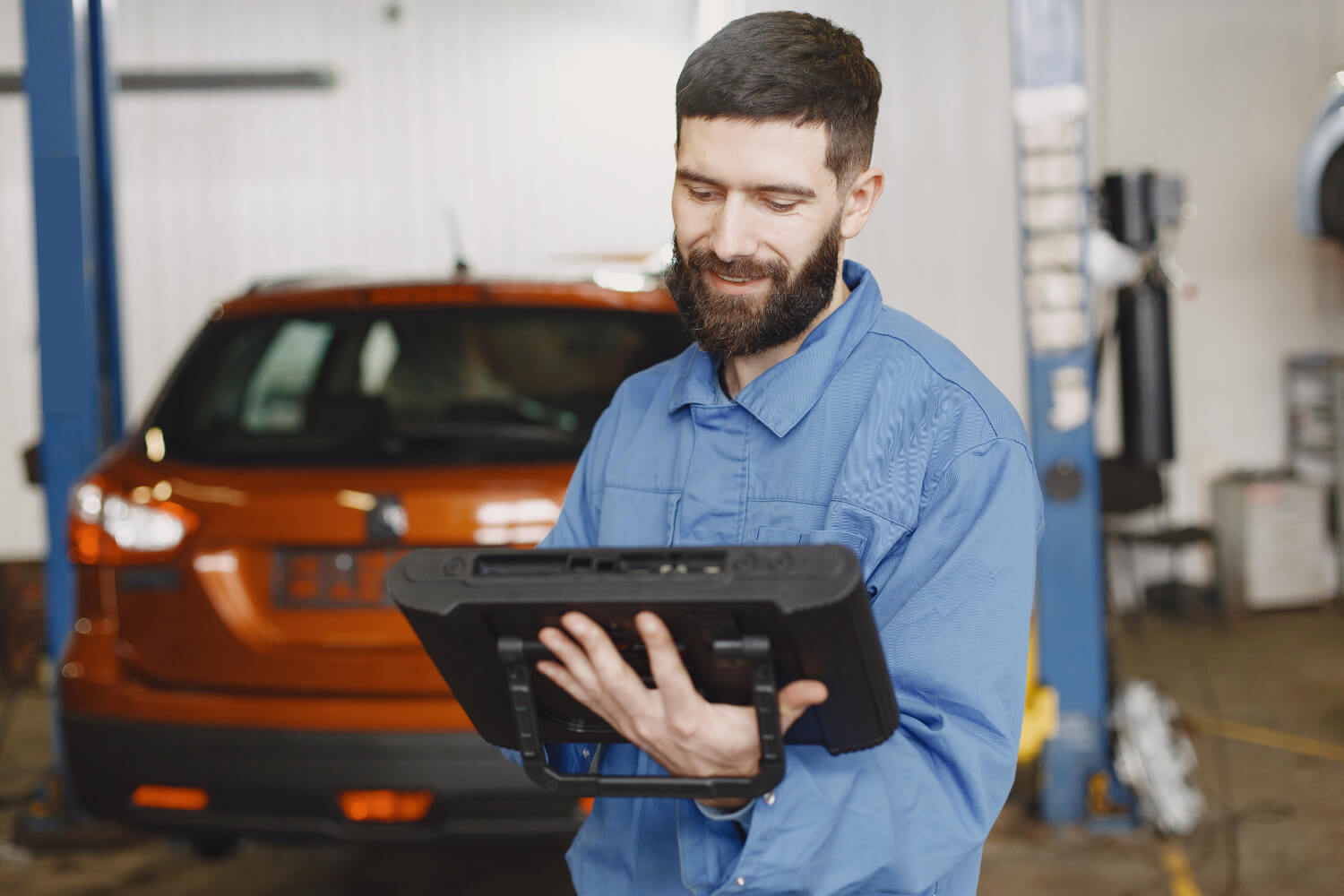
What is Quality Inspection? A Comprehensive Guide
Understand the importance of quality inspection and how Machine Vision AI is transforming manufacturing. Learn about its evolution, benefits, tools, and real-world examples.

Top Benefits of Using AI for Label Inspection in Manufacturing
Explore the key benefits of using AI for label inspection in manufacturing and understand why it is crucial in the manufacturing process.
How to Choose the Right AI Visual Inspection System for Your Needs
Learn how to select the right AI visual inspection system and explore the key factors to consider in this comprehensive guide.
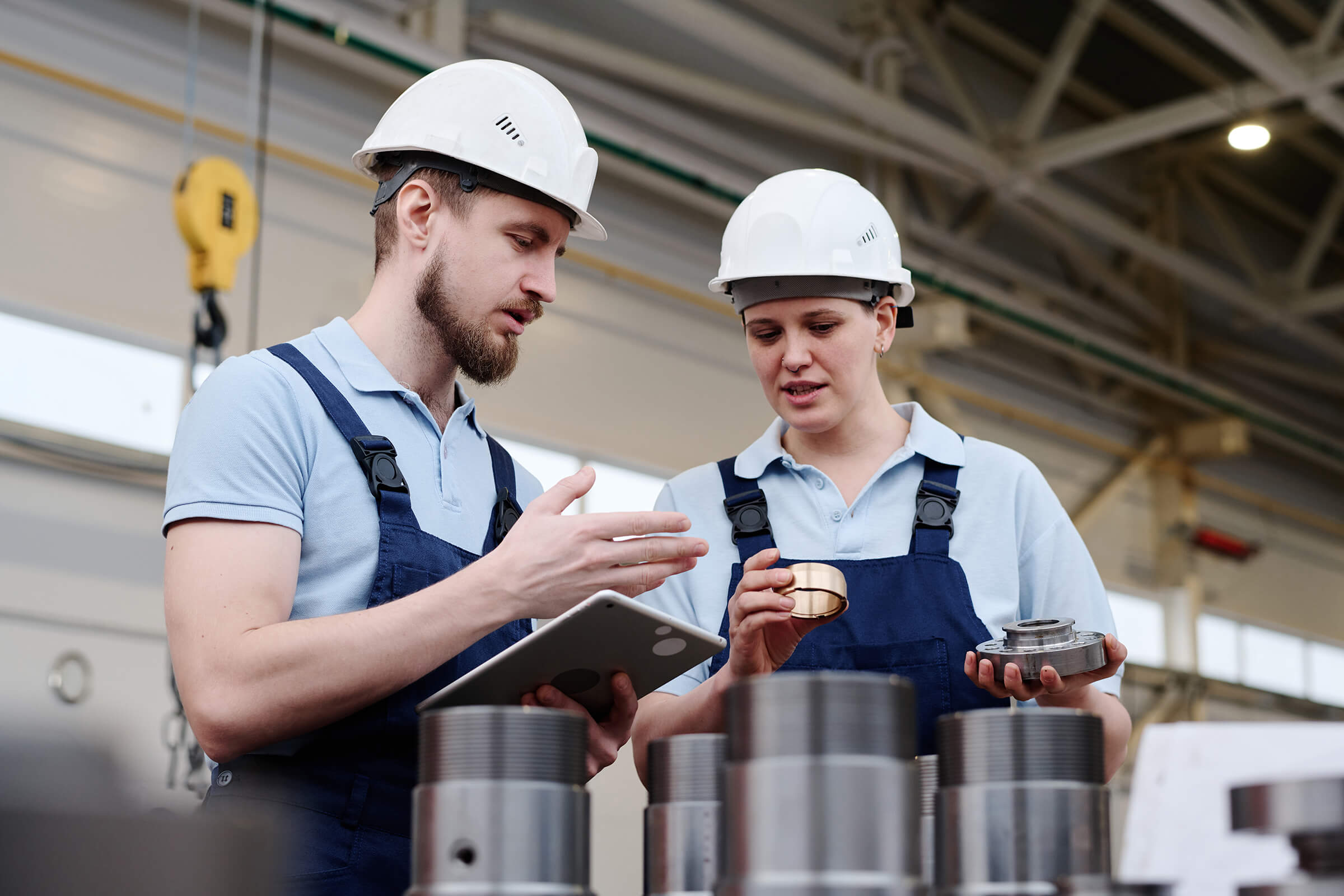
How AI-Based Visual Inspection Ensures Compliance and Upholds Standards
Learn how AI-based visual inspection ensures compliance and upholds industry standards by automating quality checks, improving accuracy, and reducing errors in manufacturing.
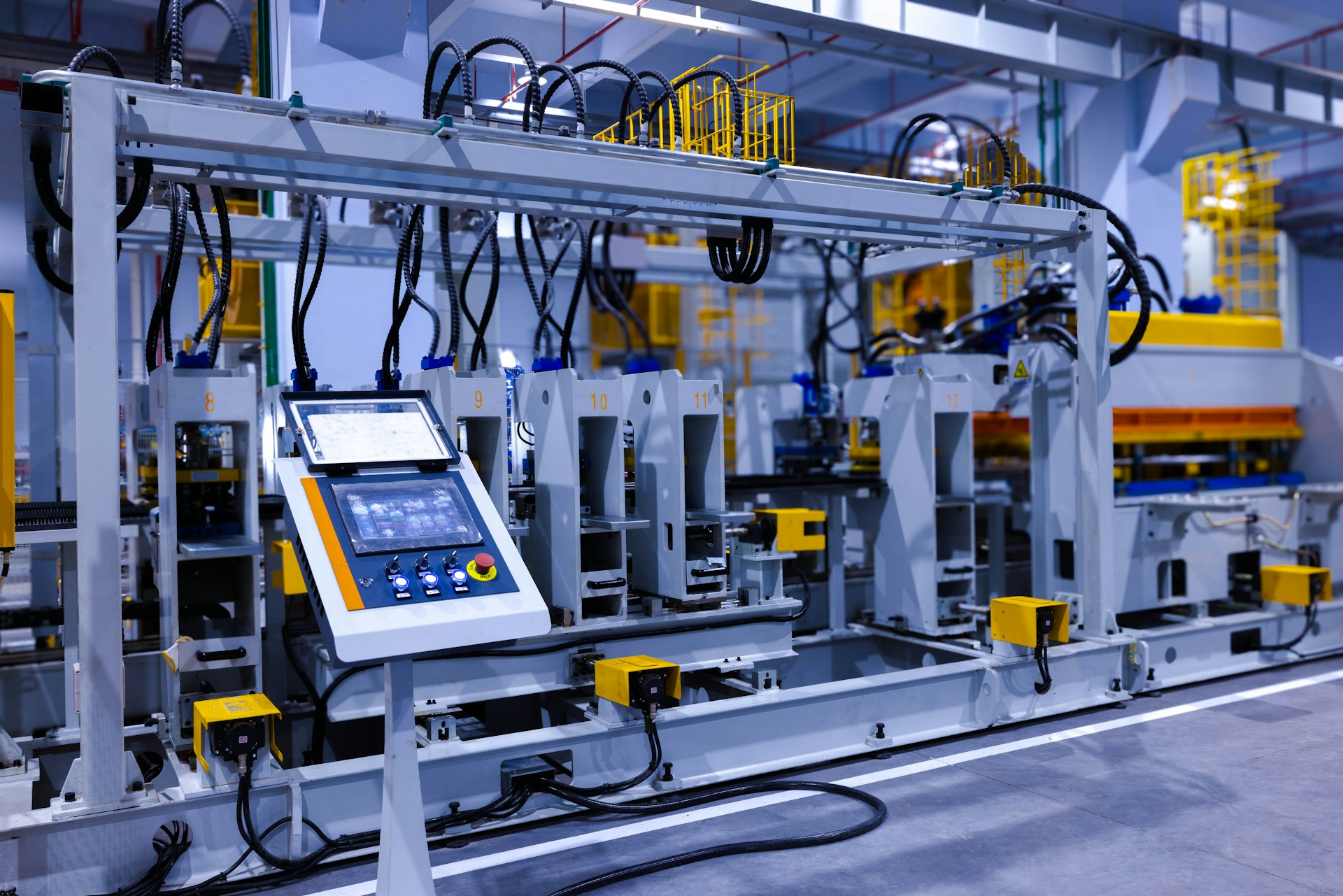
Understanding the Key Components of Machine Vision Systems
Understand the key components of machine vision systems and how they work together to enhance automation and quality control.

Applications of Machine Vision AI in Different Industries
Learn how the application of machine vision improves efficiency and quality in industries like manufacturing, automotive, aerospace and healthcare.
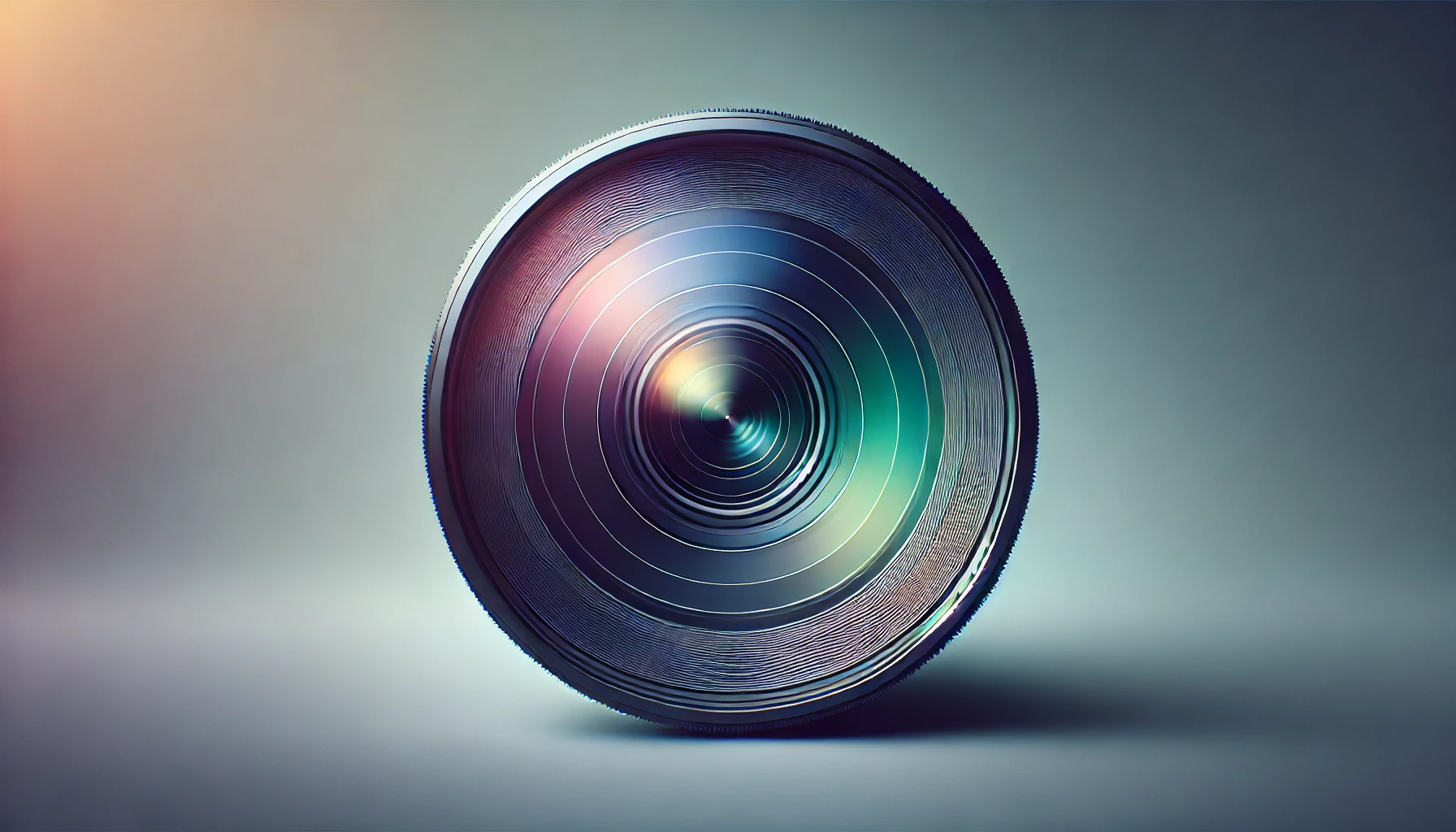
Introduction to Machine Vision: The Ultimate Guide
Explore the basics of machine vision and how it automates processes, improves accuracy, and boosts efficiency across industries.
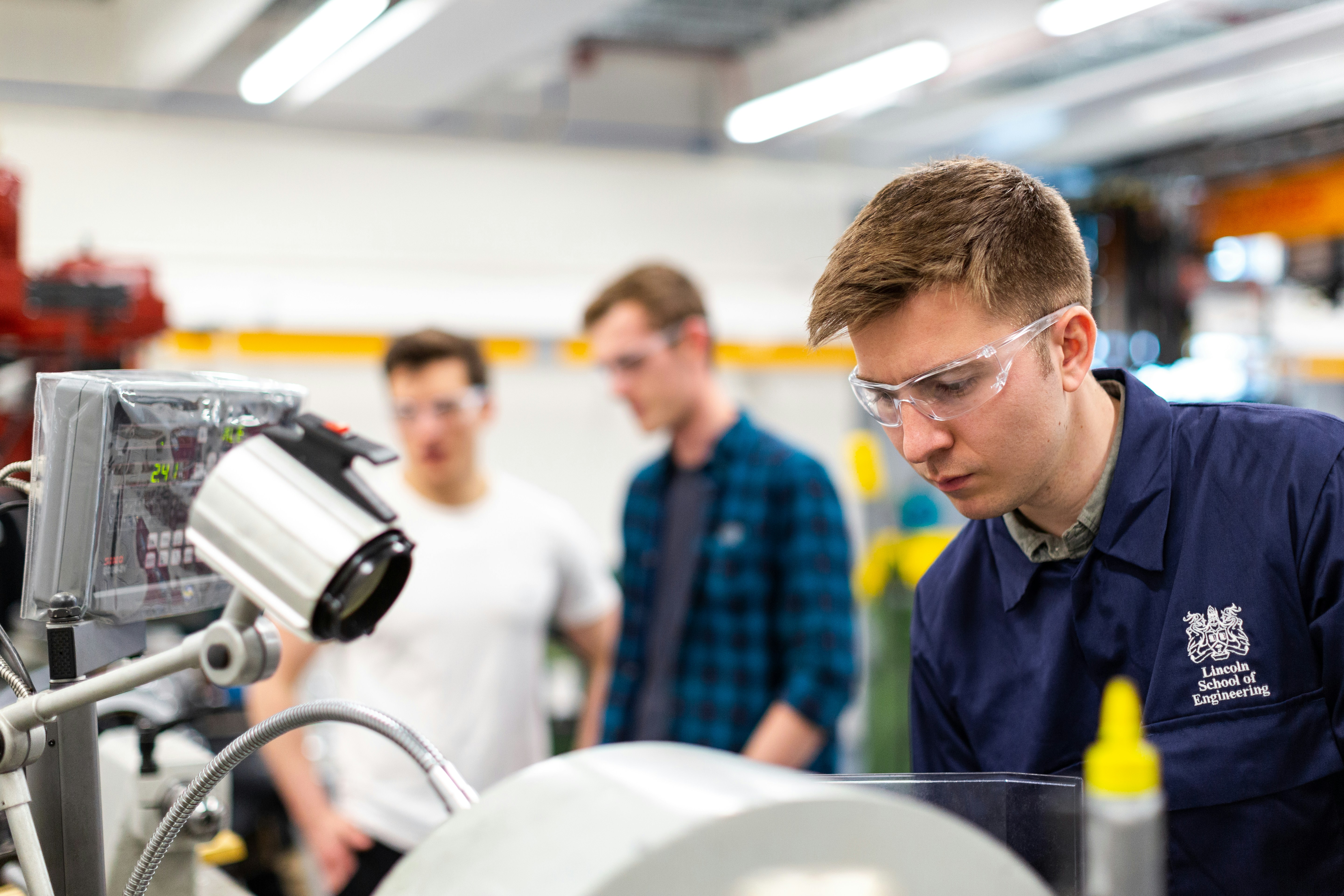
AI Quality Inspection vs. Traditional inspection Methods: A Comparative Analysis
Explore how AI-powered quality inspection outperforms traditional methods by improving speed, accuracy, and efficiency.
.jpg)
Introduction to AI Visual Inspection: Understanding the Basics
Explore the fundamentals of AI Visual Inspection and discover how it revolutionizes quality control in manufacturing.
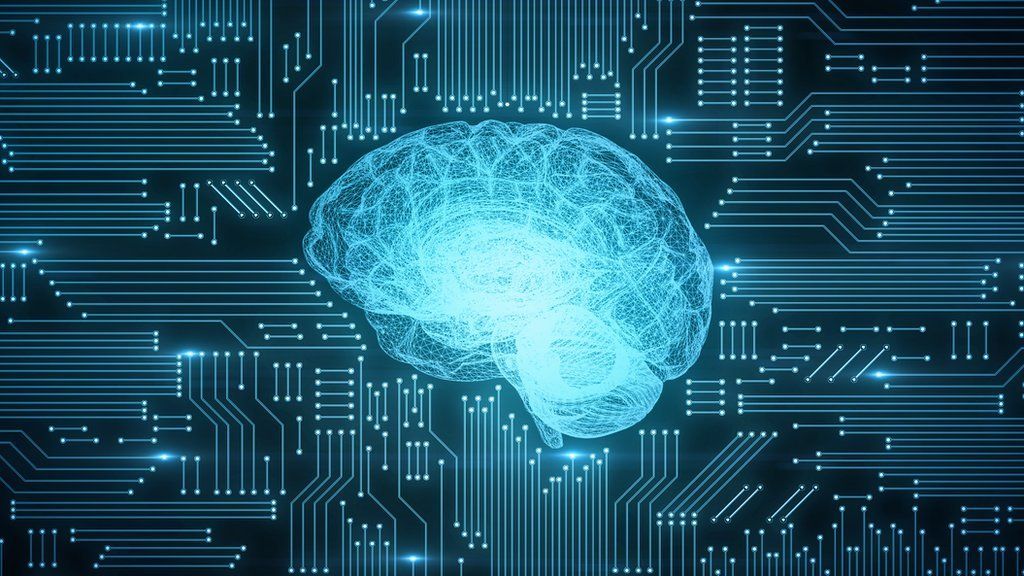
Hurdles That Enterprises Must Overcome To Adopt & Operationalize AI
AI is powerful but what are the hurdles that we need to overcome?
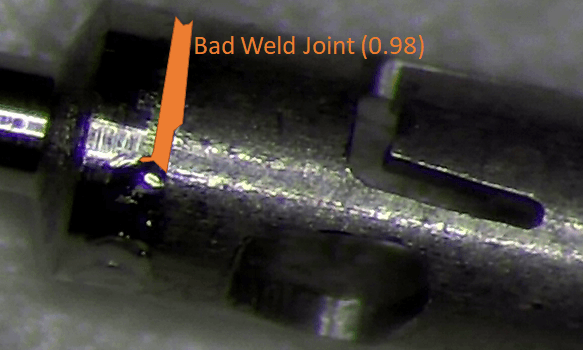
Training AI Systems For Augmenting Human Visual Inspection
Learn more about how AI can speed up visual inspection and reduce errors
.jpg)
How To Make The Most Of Your Labelling Operations?
Discover strategies to enhance your labelling operations.